Harnessing Artificial Intelligence in Cardiovascular Risk Assessment
February 02, 2022
UH Cardiovascular Medicine & Surgery | Winter 2022
From wearable technology to advanced imaging, massive quantities of data are driving innovation in precision medicine. It is estimated that the compound annual growth rate of healthcare data will reach 36 percent by 2025, outpacing that of manufacturing and financial services.1 So how can researchers harness artificial intelligence (AI) capabilities — including machine learning — to extract meaningful insight from this digital revolution?
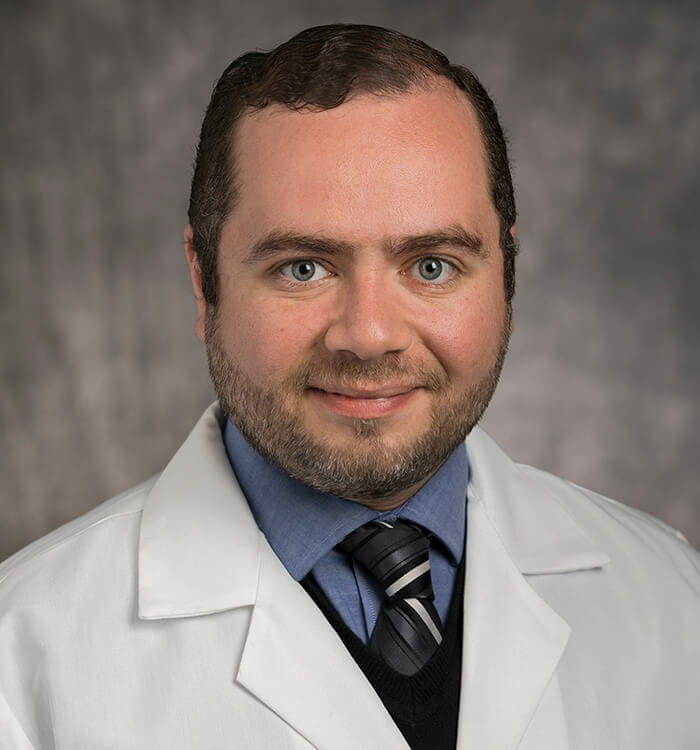
“The human mind is not able to capture the wide spectrum of data that comes to us,” says Sadeer Al-Kindi, MD, FACC, Medical Director of the Cardiovascular Phenomics Core and Co-Director of the Center for Integrated and Novel Approaches in Vascular-Metabolic Disease (UH CINEMA) within the University Hospitals Harrington Heart & Vascular Institute. “Even the smartest physicians may be able to think in one or two or three dimensions, but when it becomes hundreds of dimensions, it is impossible to perceive the patterns.”
Machine learning has emerged as a methodology to acquire, aggregate and analyze the explosion of data. In collaboration with researchers at Case Western Reserve University’s Center for Computational Imaging and Personalized Diagnostics (CCIPD) and Department of Biomedical Engineering, University Hospitals Cleveland Medical Center continues to lead the way in exploring next-generation AI applications to improve patient outcomes.
Here, Dr. Al-Kindi shares recent University Hospitals initiatives that are leveraging machine learning to classify risk and address care inequities.
Classifying risk
“People are heterogeneous, and high blood pressure or sugar levels may affect your risk differently than mine,” Dr. Al-Kindi says. “The basic concept of AI-enabled risk classification is to employ the predictive ability of machine learning by inputting large datasets of clinical information to identify patterns.”
As part of University Hospitals Harrington Heart & Vascular Institute’s cardiovascular phenomics core, experts are training algorithms to categorize patients into subgroups based on shared risk markers. “Essentially, we are finding individuals within a population who share similar risk markers,” Dr. Al-Kindi says. “We see a lot of opportunity to identify precision treatments based on the machine learning predictions.
“At the population level, we are working to integrate neighborhood-level socioeconomic status and environmental exposures (such as air pollution, traffic noise, etc.) to identify neighborhoods that have high risk for cardiometabolic disease,” he adds.
Dr Al-Kindi and his team recently presented these findings at the American Heart Association scientific sessions and showed that significant variation in cardiometabolic health metrics can be explained by socioeconomic and environmental exposures.
With respect to cardiometabolic health, Dr Al-Kindi and his team within University Hospitals Harrington Heart & Vascular Institute, along with collaborators at the Case Western Reserve University Biomedical Engineering Department, are seeking to identify novel image-based biomarkers of health. “Fat around the heart has been shown to be a risk factor for adverse cardiovascular outcomes,” Dr. Al-Kindi says. “We think that by applying machine learning to quantify and characterize epicardial fat, we will be able to identify knowable groupings of individuals who can benefit from therapies that directly impact the heart and inflammation.”
Researchers are using CT calcium score (CAC) imaging to quantify fat throughout the body, including the liver. “We see a tremendous opportunity to perform a comprehensive assessment of cardiometabolic risk beyond coronary calcifications,” Dr Al-Kindi says.
University Hospitals Harrington Heart & Vascular Institute offers no-charge coronary artery calcium scoring for community benefit and has provided over 60,000 CAC scores at no cost to patients. The study team is collecting this information as part of the CLARIFY registry.
The researchers continue to work on various applications of machine learning in cardiovascular disease. Recently, the team has published a paper on predicting the need for pacemaker implantation after aortic valve replacement.
“Our structural heart team performs a large volume of transcatheter aortic valve replacements [TAVR],” Dr. Al-Kindi says. “We’ve been integrating clinical, EKG and imaging data to identify patients who will need pacemakers in the future.”
Several investigations were presented at the 2021 American Heart Association scientific sessions, including:
- An in-depth characterization of coronary artery calcium score to improve risk stratification
- A study of how AI can help predict cardiovascular risk in patients with cancer using a standard 12-lead electrocardiogram
- A novel method for automatic quantification and characterization of epicardial fat on low-dose CT scans.
- A study of how AI can help quantify fat using low-dose CT to identify individuals with fatty liver
- Prediction of post-TAVR pacemakers using machine learning
Reducing healthcare bias
Experts at University Hospitals Harrington Heart & Vascular Institute are also using AI to bridge the gap in healthcare equity. Research shows that conventional risk calculators, primarily validated on Caucasian populations, often do not perform well in populations of different race, ethnicity or socioeconomic status.
“Our mission is to reduce bias by inputting information that better captures diversity, including clinical data, economic stressors, or environmental exposures such as air pollution,” Dr. Al-Kindi says. “Using machine learning capabilities to flag novel risk factors, we hope to predict risk with greater equity than we have in previous decades.”
“These AI approaches are making their way into clinical medicine fairly quickly,” Dr. Al-Kindi says. “The next decade or two will be about applying and validating these methodologies for different populations as patients continue to gain trust in our findings.”
For more information, email Dr. Al-Kindi at Sadeer.AlKindi@UHhospitals.org.
Contributing Expert:
Sadeer Al-Kindi, MD, FACC
Medical Director, Cardiovascular Phenomics Core
Co-Director, Center for Integrated and Novel Approaches in Vascular-Metabolic Disease
University Hospitals Harrington Heart & Vascular Institute
Assistant Professor of Medicine and Radiology
Case Western Reserve University School of Medicine